Links
Static search trees: 40x faster than binary search
Table of Contents 1 Introduction 1.1 Problem statement 1.2 Motivation 1.3 Recommended reading 1.4 Binary search and Eytzinger layout 1.5 Hugepages 1.6 A note on benchmarking 1.7 Cache lines 1.8 S-trees and B-trees 2 Optimizing find 2.1 Linear 2.2 Auto-vectorization 2.3 Trailing zeros 2.4 Popcount 2.5 Manual SIMD 3 Optimizing the search 3.1 Batching 3.2 Prefetching 3.3 Pointer arithmetic 3.3.1 Up-front splat 3.3.2 Byte-based pointers 3.3.3 The final version 3.4 Skip prefetch 3.5 Interleave 4 Optimizing the tree layout 4.1 Left-tree 4.2 Memory layouts 4.3 Node size \(B=15\) 4.3.1 Data structure size 4.4 Summary 5 Prefix partitioning 5.1 Full layout 5.2 Compact subtrees 5.3 The best of both: compact first level 5.4 Overlapping trees 5.5 Human data 5.6 Prefix map 5.7 Summary 6 Multi-threaded comparison 7 Conclusion 7.1 Future work 7.1.1 Branchy search 7.1.2 Interpolation search 7.1.3 Packing data smaller 7.1.4 Returning indices in original data 7.1.5 Range queries 7.1.6 Sorting queries 7.1.7 Suffix array searching In this post, we will implement a static search tree (S+ tree) for high-throughput searching of sorted data, as introduced on Algorithmica. We’ll mostly take the code presented there as a starting point, and optimize it to its limits. For a large part, I’m simply taking the ‘future work’ ideas of that post and implementing them. And then there will be a bunch of looking at assembly code to shave off all the instructions we can. Lastly, there will be one big addition to optimize throughput: batching.
GitHub - GAIR-NLP/OpenResearcher
Contribute to GAIR-NLP/OpenResearcher development by creating an account on GitHub.
Chain of Thought Empowers Transformers to Solve Inherently Serial Problems
Instructing the model to generate a sequence of intermediate steps, a.k.a., a chain of thought (CoT), is a highly effective method to improve the accuracy of large language models (LLMs) on arithmetics and symbolic reasoning tasks. However, the mechanism behind CoT remains unclear. This work provides a theoretical understanding of the power of CoT for decoder-only transformers through the lens of expressiveness. Conceptually, CoT empowers the model with the ability to perform inherently serial computation, which is otherwise lacking in transformers, especially when depth is low. Given input length $n$, previous works have shown that constant-depth transformers with finite precision $\mathsf{poly}(n)$ embedding size can only solve problems in $\mathsf{TC}^0$ without CoT. We first show an even tighter expressiveness upper bound for constant-depth transformers with constant-bit precision, which can only solve problems in $\mathsf{AC}^0$, a proper subset of $ \mathsf{TC}^0$. However, with $T$ steps of CoT, constant-depth transformers using constant-bit precision and $O(\log n)$ embedding size can solve any problem solvable by boolean circuits of size $T$. Empirically, enabling CoT dramatically improves the accuracy for tasks that are hard for parallel computation, including the composition of permutation groups, iterated squaring, and circuit value problems, especially for low-depth transformers.
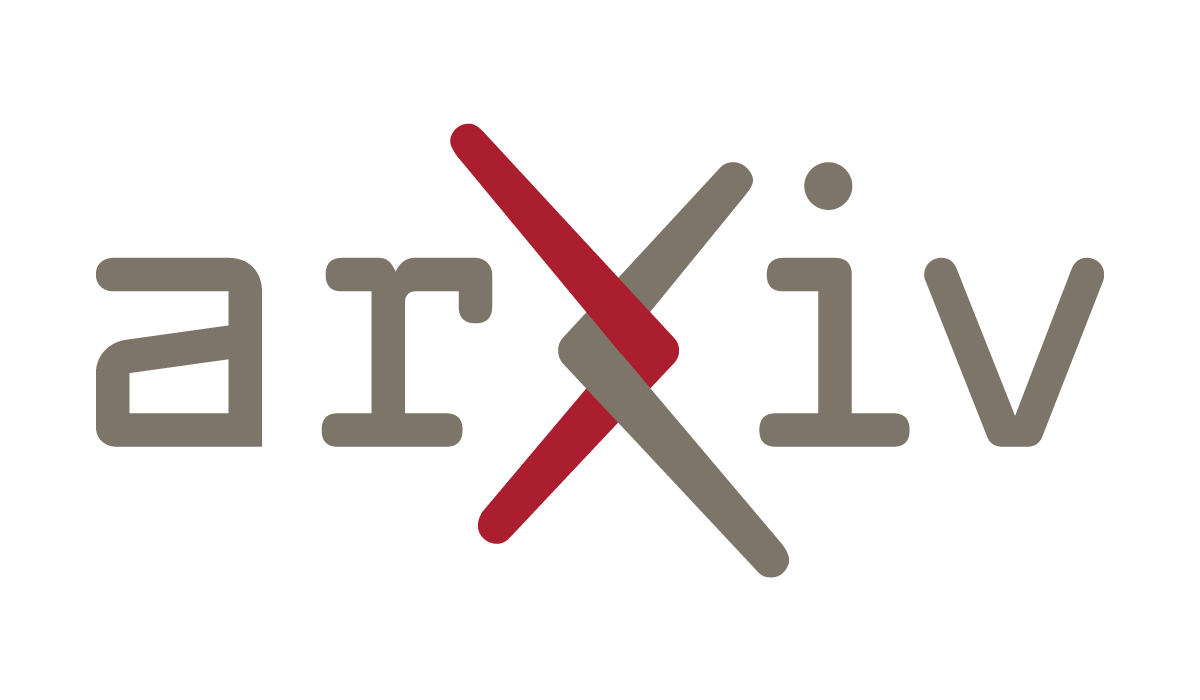
Prompt Engineering Guide – Nextra
A Comprehensive Overview of Prompt Engineering
GitHub - OSU-NLP-Group/HippoRAG: HippoRAG is a novel RAG framework inspired by human long-term memory that enables LLMs to continuously integrate knowledge across external documents. RAG + Knowledge Graphs + Personalized PageRank.
HippoRAG is a novel RAG framework inspired by human long-term memory that enables LLMs to continuously integrate knowledge across external documents. RAG + Knowledge Graphs + Personalized PageRank.…
Quantum error correction below the surface code threshold

GitHub - SakanaAI/AI-Scientist: The AI Scientist: Towards Fully Automated Open-Ended Scientific Discovery 🧑🔬
The AI Scientist: Towards Fully Automated Open-Ended Scientific Discovery 🧑🔬 - SakanaAI/AI-Scientist
A ConvNet for the 2020s
The “Roaring 20s” of visual recognition began with the introduction of Vision Transformers (ViTs), which quickly superseded ConvNets as the state-of-the-art image classification model. A vanilla ViT, on the other hand, faces difficulties when applied to general computer vision tasks such as object detection and semantic segmentation. It is the hierarchical Transformers (e.g., Swin Transformers) that reintroduced several ConvNet priors, making Transformers practically viable as a generic vision backbone and demonstrating remarkable performance on a wide variety of vision tasks. However, the effectiveness of such hybrid approaches is still largely credited to the intrinsic superiority of Transformers, rather than the inherent inductive biases of convolutions. In this work, we reexamine the design spaces and test the limits of what a pure ConvNet can achieve. We gradually “modernize” a standard ResNet toward the design of a vision Transformer, and discover several key components that contribute to the performance difference along the way. The outcome of this exploration is a family of pure ConvNet models dubbed ConvNeXt. Constructed entirely from standard ConvNet modules, ConvNeXts compete favorably with Transformers in terms of accuracy and scalability, achieving 87.8% ImageNet top-1 accuracy and outperforming Swin Transformers on COCO detection and ADE20K segmentation, while maintaining the simplicity and efficiency of standard ConvNets.
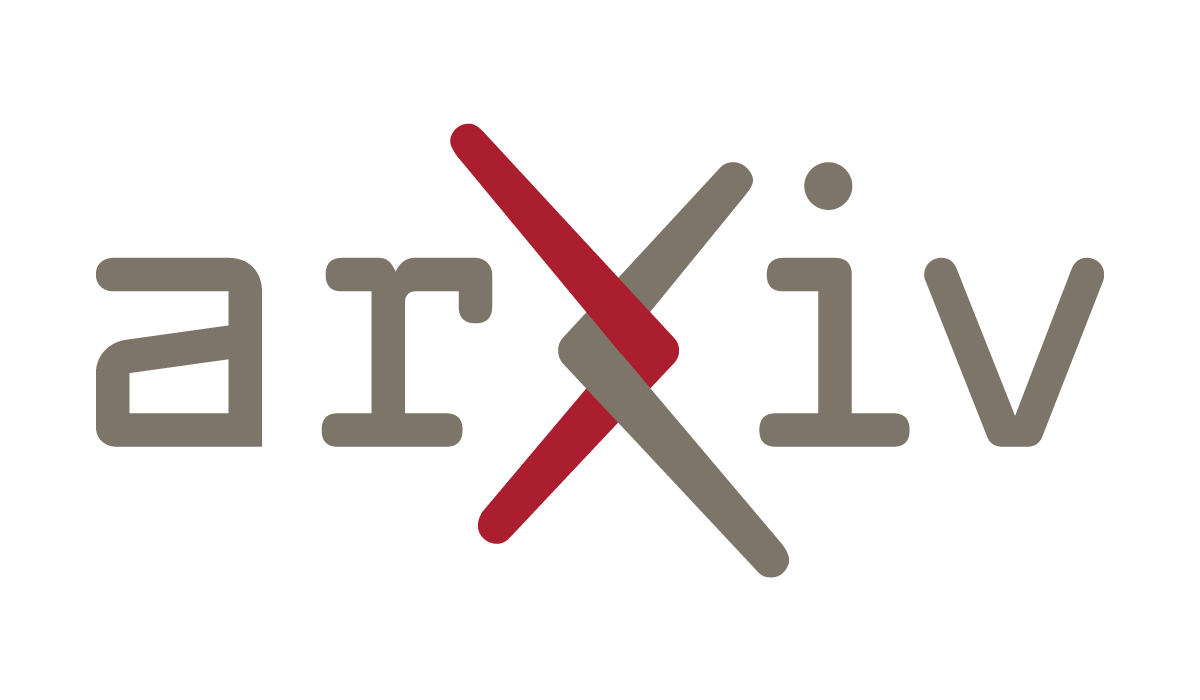
Building A Generative AI Platform
After studying how companies deploy generative AI applications, I noticed many similarities in their platforms. This post outlines the common components of a generative AI platform, what they do, and how they are implemented. I try my best to keep the architecture general, but certain applications might deviate. This is what the overall architecture looks like.
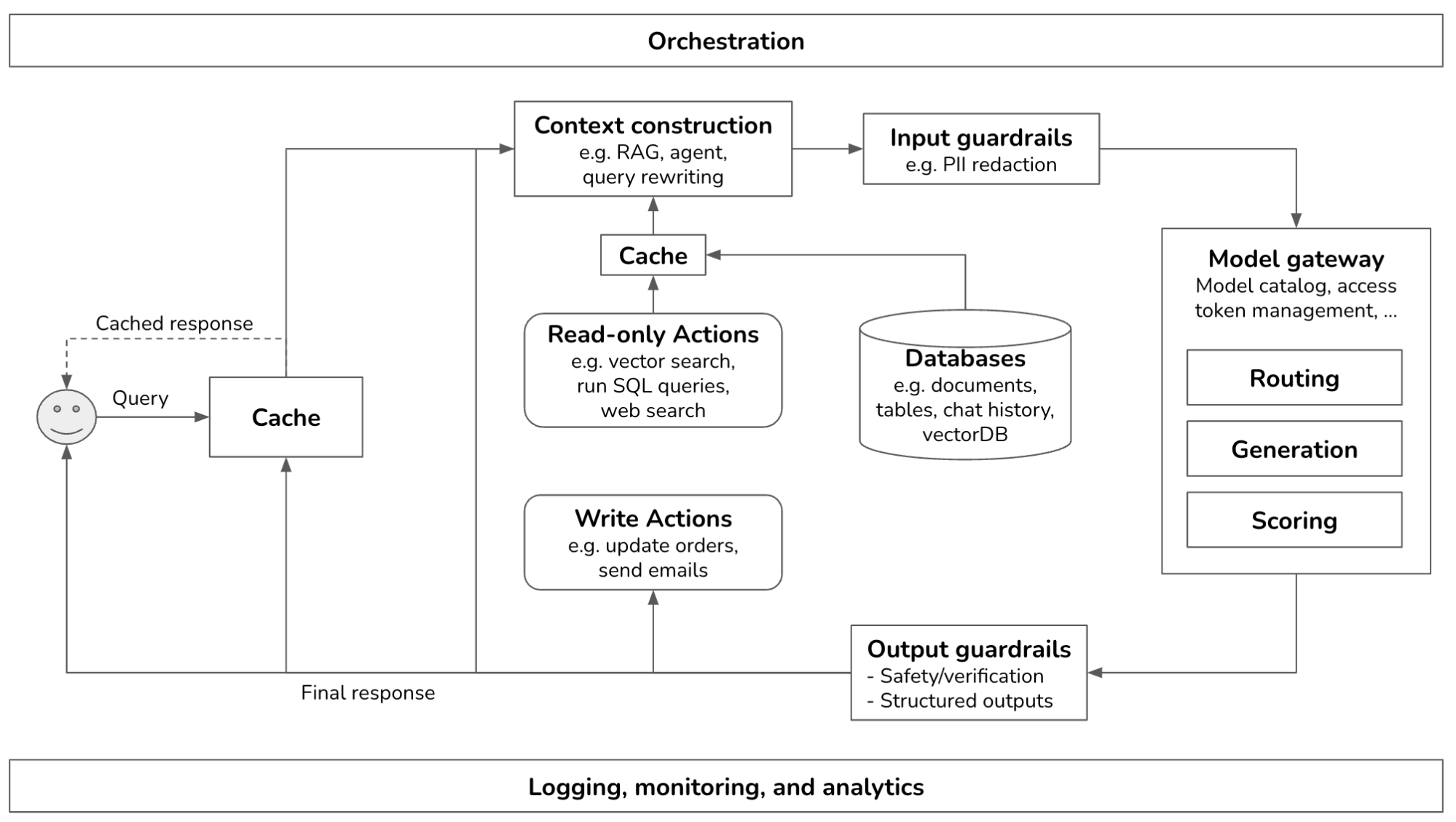